Computational Models and Machine Learning Improve Cardiac Diagnostics
A tremor in the breast and the feeling as if the heart would stop beating – such an extrasystole often is harmless, but painful for persons affected. Extrasystoles are mostly caused by additional beats of the heart. They cause fear and sometimes may increase the risk of life-threatening arrhythmias. At Karlsruhe Institute of Technology (KIT), researchers are working on facilitating and improving the diagnosis and therapy of cardiac diseases through computer modeling.
Stephanie Appel is one of the researchers working in the Computational Cardiac Modeling Group at KIT’s Institute of Biomedical Engineering (IBT). The group has set itself the goal of mathematically translating heart function and realistically reproducing it in a computer model, thereby contributing to the improvement of medical care. Stephanie Appel’s project focuses on atrial fibrillation, a type of cardiac arrhythmia resulting from an arrhythmic propagation of electric pulses in the atria. Such pulses can no longer contribute to the pumping function of the heart. “In my work, I try to accurately simulate the excitation propagation in the patients’ hearts. Using patient-specific data, I create individual computer models and develop methods to characterize the cause of the disorder more precisely,” Appel says.
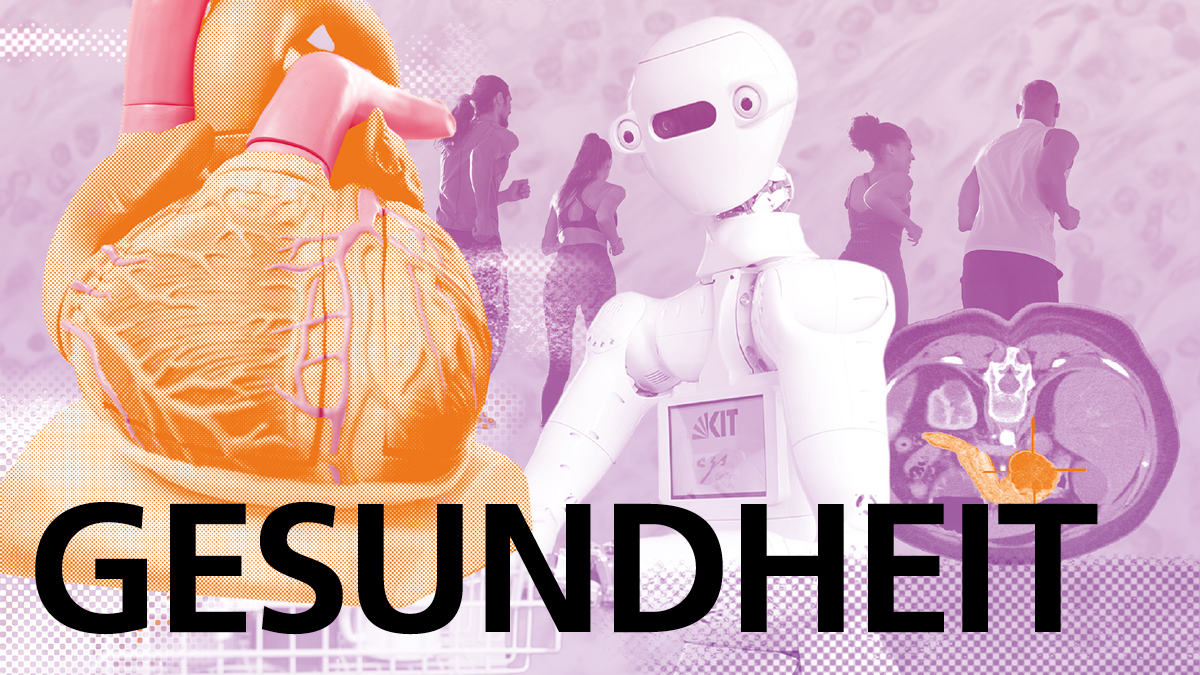
Issue 2024/2 of the lookKIT research magazine deals with methods and technologies to improve health care.
To the MagazineTreating Atrial Fibrillation without Scars
Appel’s doctoral project is part of the collaborative research center “Wave Phenomena” at KIT. Appel will input new parameters in an existing computer model and optimize the latter to come up with a new therapy of atrial fibrillation. In current ablation therapy, abnormal tissue is targeted and destroyed using cold and high-frequency current injected by a catheter. However, this leaves behind scarred tissue. With the new findings, the researchers hope to be able to provide pacemakers that are able to interrupt atrial fibrillation with precisely timed and spatially coordinated impulses. This would prevent permanent modification of heart muscle tissue and, hence, the risks of perforation or scarring.
Dr. Axel Loewe, Head of the Working Group at IBT, points out that the model developed by the researchers models different functions of the heart with the help of mathematical equations. “One focus lies on electrophysiology of the heart. Cardiac rhythm is coordinated by electrical activity that is translated into mathematical equations. These describe the different biological levels in the heart down to the smallest building blocks that open and close depending on the electrical voltage.”
Using Digital Twins for Successful Therapy
A study of last year reveals the success of IBT’s research. In collaboration with the University Heart Center Freiburg-Bad Krozingen, the Group compared the potential of the new modeling method with current therapies. Based on data of 29 persons, the researchers produced a digital twin of the hearts of the patients in order to detect the causes of extrasystoles.
“We found that accurate localization of connective tissue deposits in the atria is crucial to the success of personalized therapy. If the therapy, which electrically isolates the heart tissue, is applied to precisely these areas, cardiac arrhythmia can be terminated. With conventional therapy methods, these regions disturbing the rhythm can only be roughly localized. The therapy then no longer works in a third of patients,” Loewe explains. With their new method, researchers could accelerate medical interventions, reduce risks, and improve results, the electrical engineer points out.
Combination of Scientific Fundamentals and Artificial Intelligence
KIT’s working group has increased the accuracy of its computer models through machine learning. As clinical patient data is poorly available and often not systematically recorded in Germany, the researchers have trained their application with simulated data. Loewe explains the approach using atrial fibrillation as an example: “Conventional ablation therapy is successful in about 50% of the patients. However, it is difficult to predict which patients can hope for success. We calculated more than 1000 episodes of atrial fibrillation in a computer model and carried out virtual ablations. With these simulated data, we trained a machine learning method.”
To assess the model, the results were compared with real datasets. Modeling was found to increase the potential of medical interventions: In 83 percent of all clinical cases, the researchers identified in advance those patients that would not benefit directly from an ablation therapy, just by using an ECG. “This is a fascinating process. Synergy of computer models that are based on the basic laws of physics and biology and the increasing integration with machine learning and artificial intelligence will become more and more important in medicine in the future,” Loewe says.
Heike Marburger, August 26, 2024
Translated by: Dipl.-Übers. Maike Schröder